Contributers:
Rashi Shrishrimal (201301203)
K S Chandra Reddy (201505544)
Naman Singhal (201330072)
Project Guide
Dr. Vasudev Varma
Priya Radhakrishnan
Abstract
The 2012 ACM Computing Classification System has been developed as a poly-hierarchical ontology that can be utilized in semantic web applications. A research paper in acm digital library should ideally have the categories associated it. Our goal is to automate the process so as to predict the most appropriate category, the research paper lies in.
Introduction
The semantic annotation of documents is an additional advantage for retrieval, as long as the annotations and their maintenance process scale well.
With the rapid growth of the information amount available online and in databases, search engines play an important role within eLearning, since they can support the learner in looking for the needed information for his learning, training or teaching process. However, these information extraction systems are based on terms indexation without taking into account neither the semantics of learning information’s contents nor the context. In general, users usually enter keywords into search engines, that are based on terms indexation without taking into account neither the semantics of the pedagogical content nor the context.
Here, we discuss three approaches to go about the problem, namely basic cosine similarity, latent dirichlet allocation and labeled lda. As the work progresses we see how we get better results and the best with the labeled lda.
Problem Statement
Given an abstract of a research paper, we need to find the category that it fits into.The 2012 ACM Computing Classification System has been developed as a poly-hierarchical ontology that can be utilized in semantic web applications.The complete classification tree can be found here.
Datasets
- A private dataset of the SIEL lab of IIIT-Hyderabad.
- Title
- Author
- Country of Authors
- Year of Publication
- Conference
- Categories
- Abstract (Only for some papers)
- Wiki dataset
- dblp dataset
Description : For every research paper there are the following fields:
Description : For all the acm categories a dataset was build having the summary of the wikipedia page (The First section of the wikipedia document).
Description : For initial tasks, this dataset was used to train the lda model. Though this is not useful for the final proposition of the model.
Our Solution
- Cosine Similarity
In our approach we took the wikipedia summary of each of the categories as separate documents and measured the cosine similarity of the test document with each of them and assigned the category whose wikipedia document was most similar.
-
Latent Dirichlet Allocation
In our approach, we used LDA (topic modelling algorithm) to cluster documents and assign a category to each cluster depending on the cluster in which the wikipedia document of the category is matching the most. Then put the test document on same model and assigned the category depending on the cluster to which test document belonged and the category assigned to that cluster.
-
Labeled Latent Dirichlet Allocation + Doc2Vec
Accuracy can only be improved by supervised topic modelling i.e. by labelling the documents with topics and taking care of the semantic distance between the research paper and the categories.
Results
-
The simple cosine similarity approach could not encompass the semantic part of the documents and hence the categories. The Keyword matching though was acceptable. It is because no latent idea was incorporated in the model. 5% for the categories and 30% for the keywords.
-
Latent Dirichlet Allocation was able to incorporate the idea of semantic annotation as latent terms were found. But, then also the latent terms it predicts are not guaranteed to match the actual categories due to its unsupervised nature. The cluster has many wiki documents and hence the acm categories. The downsizing in the number of clusters resulted in the problem. The accuracy was less than 15%.
-
Labeled lda : This is the most sensible algorithm for this type of problem. The results show the MAP value of 59.31% which we believe is good provided the type of data.
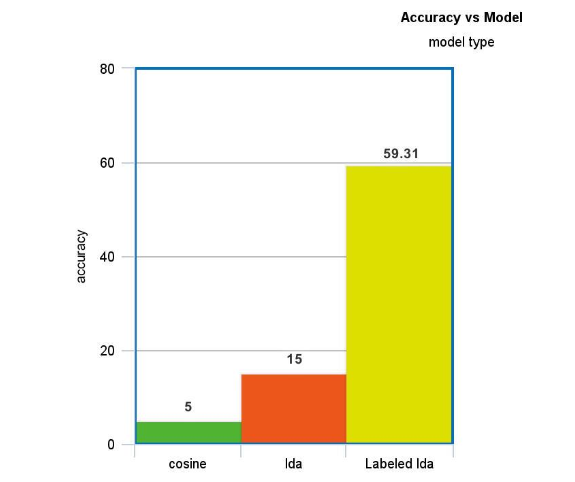
Comparision
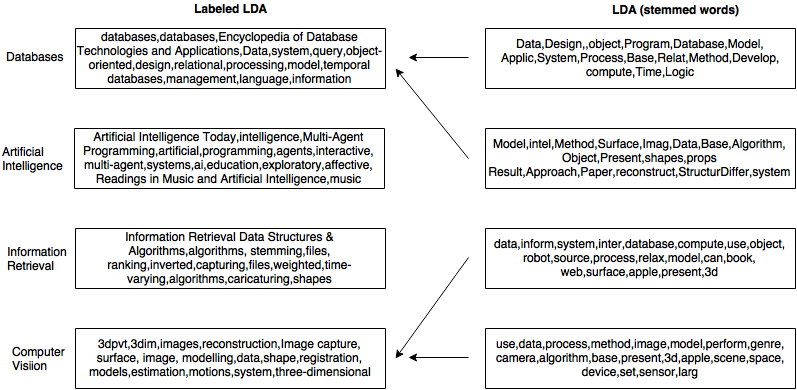
If we take top twenty words from each category it can be seen that labeled LDA has greatly increased the number of relevant words in the cluster, thereby affecting and increasing the accuracy. It can also be seen that cluster belonging to a particular category given by LDA has a lot of noise or say words which can be related to other categories as well, thereby it is less reliable to start with and so can’t be expected to accurately classify test data.
Observation
We started off with Cosine Similarity, which doesn’t cash-in semantic similarity in classifying test data. Latent Dirichlet Allocation though takes semantics into account, it doesn’t make the best use of training data, where label of each training document can’t be used to train the model. Labeled LDA improved on LDA, by taking in category each document belongs to as well during training, thereby providing most relevant set of words per category, so does a better job in categorizing test data.